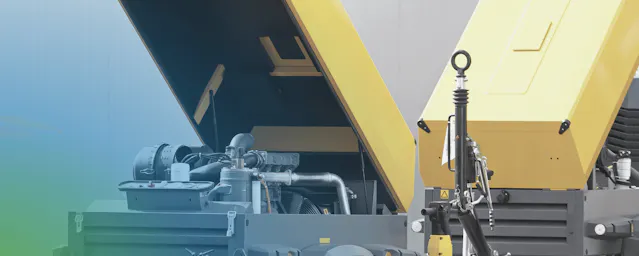
Successfully using AI in the context of smart products
AI makes it possible
Smart products are inherently equipped with a certain basic intelligence without which they cannot fulfill their function.
With the addition of artificial intelligence, however, completely new use cases can be implemented that offer added value for manufacturers and operators - e.g:
- AI-supported optimization of a machine's operating parameters - for quality assurance, for example, or generally for efficient, sustainable machine operation. AI opens up new possibilities here, as optimal operation requires both a deep understanding of the machine (which only the manufacturer has) and knowledge of the individual operating situation (upstream or downstream process steps, spatial environment of the machine, etc.), which only the operator has. It is generally not possible to bring these two areas of expertise together in a concentrated manner, but an AI-supported analysis can be the solution to this problem.
- Predictive maintenance for products in customer use: This also involves evaluating and interpreting individual health, performance and process data from machines/products operated by the customer in real time - a complexity that is difficult or impossible to map without AI.
Both scenarios can be initiated by both the operator and the manufacturer and can be technically mapped both embedded and in the cloud.
AI is therefore an effective means of addressing complex problems - but a complex problem does not mean that the implementation must also be complex. So the question arises as to why, in practice, AI projects often fail in companies or only deliver inadequate results?
There is actually no reason for this. Because with a structured approach such as the Data Science Life Cycle and solid groundwork, AI projects can also be implemented in a targeted and efficient manner.
This is shown in practice
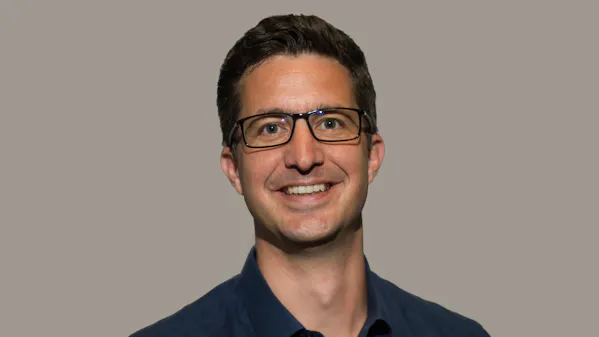
Andreas Oyrer, CEO BLUE-ZONE GmbH
Data Science Life Cycle
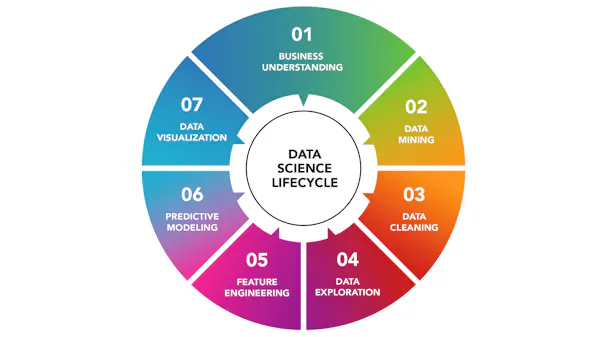
In practice, it will often be necessary to carry out a second data mining (with an improved data basis) after data cleaning or even to take another look at the business understanding or the desired business case - especially if it turns out that the goal cannot be achieved with the existing data and no additional data can be acquired through further sensor technology.
Again, the more careful the work in phase 1, the less need there will be for subsequent adjustments. BLUE-ZONE GmbH has extensive expertise and project experience, particularly in phases 1-3, from which our customers benefit in the long term.
According to Anaconda's Data Science Report, an average of 38% of the total costs of an AI project are incurred for data preparation and data cleansing alone. However, there are also upstream activities such as acquiring the data and gaining an understanding of the case. At the same time, this is where the course is set for how targeted and efficient the project will be in the future: If the foundation has been laid properly, less reworking, corrections etc. will be required in the later phases.
As described above, the success of an AI project and the overall effort involved depend to a large extent on a clear foundation being laid in phases 1 and 1-3.
Our offer for you
Invest 60 minutes in a non-binding innovation exchange to gain an initial basic understanding of how to set up an AI project and lay the foundations for the desired business case - and benefit from efficient and targeted project progress in the future.
Your contact person
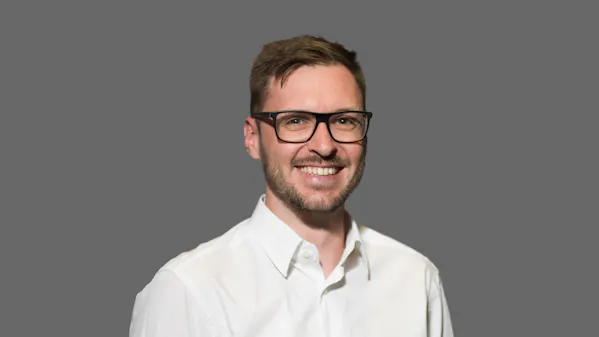